Welcome to the OMS (Optimization on Measures Spaces) project.
ANR JCJC start 2018 – end 2020.
How to optimize a structured measure? Such objects can model sparse spikes in super-resolution, sampling trajectories in magnetic resonance imaging, or contours in images… We aim at developing novel theoretical and computational tools to address this issue and to apply them on modern challenging applications.
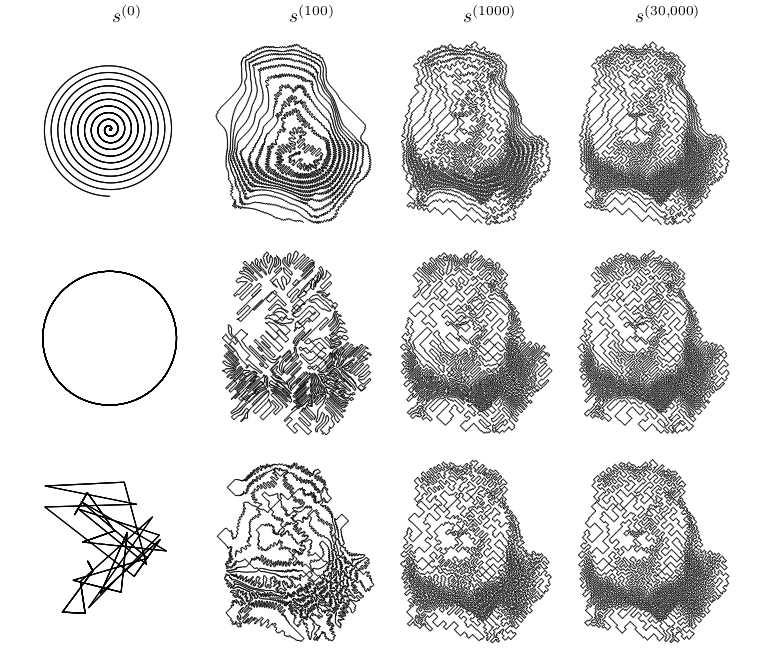
Dynamics of our projection algorithm: starting from any curve, the sequence of measures converges to the image of a lion.
Extended abstract:
From quantisation to sketching for large-scale learning or infinite dimensional inverse problems, to name but a few, many different problems may be cast as an optimisation problem where the target is a measure.
An important special case is measure approximation, which amounts to computing a projection. A simple instance of this problem is half-toning, which is the cornerstone of most printing digital inkjet devices. The image may be seen as the target measure, and the authorised measures are the collections of N atoms with weight 1/N. More generally, we may want to minimise any function on a subset of the Radon probability measures on a d-dimensional manifold.
Many different algorithms have been devised to solve instances of these problems. They range from Galerkin approaches, to greedy algorithms, lifting schemes, or Lasserre hierarchies. These approaches work well for low complexity problems but are either far too slow or loose for some practical applications targeted in this project.
In former work, we have leveraged the fact that any subset of Radon probability measures is a limit in Hausdorff distance of sets of measures with N atoms. This is especially natural for curves seen as pushforward measures, or more generally for sets of structured submanifolds. We have then developed a versatile algorithm to solve the resulting discretised optimisation problem.
The main benefit is that there are only Nd variables, so that the complexity scales linearly with the dimension. Moreover the approximation rate of measures with N atoms is often much better than more traditional discretisation schemes. For instance, since we do not work on a grid, the atoms can be precisely pinpointed.
The drawback is that the optimisation problem is highly non-convex. Hence, there is little hope of finding the global minimum, in general. The century-old Thomson problem, which is a Smale problem, is but a special case. We are therefore interested in critical points and local minima.
The project aims at improving underlying tools for our algorithms, giving general theoretical guarantees, and applying the techniques to concrete applications.
First, we want to find efficient discretisations for several specific sets of measures. Efficient both as approximating quickly and as computationally tractable. We also need to improve and generalise geometric tools, such as the Non-Uniform Fast Fourier Transform, and the Laguerre diagrams. In particular, we would like to develop similar tools for curves rather than points.
Second, we want to prove convergence of our algorithms, and develop new ones if need be. An intriguing and more general theoretical question is to understand when an optimisation algorithm will converge to a “good” suboptimal solution despite non-convexity, as it seems to happen in practice.
Finally, we will apply our tools to the optimisation of acquisition paths in Magnetic Resonance Imaging and the reconstruction of super-resolved images in microscopy, with an eye on commercialisation. We will explore other applications such as sparse spike deconvolution and galaxy filament detection.
The project involves researchers all in Toulouse, with diverse and complementary expertise. The Principal Investigator (PI) Jonas Kahn (CNRS, IMT) covers a wide spectrum of mathematics and their applications. He belongs to and may promote interactions with the newly-founded “équipe-projet” on machine learning at IMT as well as the bio-imaging team PRIMO at ITAV. Pierre Weiss (CNRS, ITAV) and Frédéric de Gournay (IMT) are also members of this team. Their work on MRI and transportation distances is the origin of the project. They specialise in numerical analysis and implementation of algorithms. Jérôme Bolte (TSE) is a known specialist on the theory of optimisation. Léo Lebrat started his PhD student in 2016/09 under the supervision of Jonas Kahn and Frédéric de Gournay.